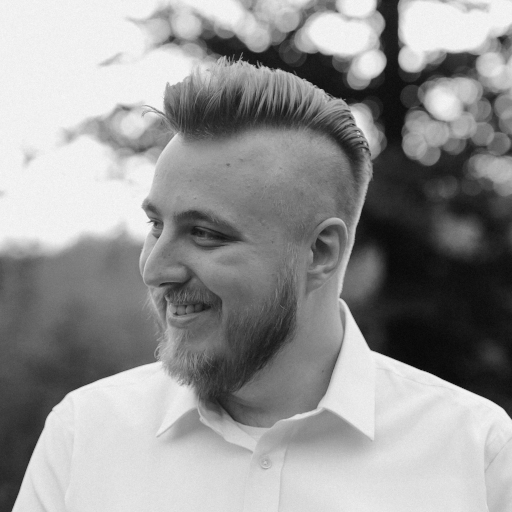
Christopher Schymura
I am an experienced data scientist and machine learning researcher, enjoying to build data-driven solutions for real-world applications. My interests particularly focus on combining probabilistic models with deep learning techniques to develop end-to-end trainable systems with meaningful uncertainty estimates. Currently, I am working as a data scientist at Bayer Crop Science. I am also a co-founder of the Data Science Kitchen, a non-profit group which promotes open source research and regularly participates in data science challenges.
Before joining Bayer, I was a data scientist in the Cognitive Solutions team at Evonik Digital GmbH and a postdoctoral researcher in the Cognitive Signal Processing Group at Ruhr University Bochum. My postdoctoral research focused on fusing classical probabilistic models with modern deep learning approaches for applications in audiovisual signal processing. I successfully conducted a joint research project with NTT Communication Science Labs, which was geared towards deep learning models for probabilistic inference in multimodal time-series signals.
I obtained a PhD degree in Electrical Engineering and Information Technology from Ruhr University Bochum, advised by Dorothea Kolossa. During my time as a doctoral researcher at the Cognitive Signal Processing Group, I focused on probabilistic graphical models for multimodal data fusion. A central aspect of my PhD thesis was the derivation of the Dynamic Stream Weight Kalman Filter - a model for recursive Bayesian estimation in dynamical systems with multimodal observations, where time-varying uncertainty in different sensor inputs is explicitly taken into account. Other aspects of my PhD research included causal inference with application to acoustic signal processing tasks and Bayesian methods for robot audition, where the latter was primarily tackled within the Two!Ears research consortium.
Before joining Bayer, I was a data scientist in the Cognitive Solutions team at Evonik Digital GmbH and a postdoctoral researcher in the Cognitive Signal Processing Group at Ruhr University Bochum. My postdoctoral research focused on fusing classical probabilistic models with modern deep learning approaches for applications in audiovisual signal processing. I successfully conducted a joint research project with NTT Communication Science Labs, which was geared towards deep learning models for probabilistic inference in multimodal time-series signals.
I obtained a PhD degree in Electrical Engineering and Information Technology from Ruhr University Bochum, advised by Dorothea Kolossa. During my time as a doctoral researcher at the Cognitive Signal Processing Group, I focused on probabilistic graphical models for multimodal data fusion. A central aspect of my PhD thesis was the derivation of the Dynamic Stream Weight Kalman Filter - a model for recursive Bayesian estimation in dynamical systems with multimodal observations, where time-varying uncertainty in different sensor inputs is explicitly taken into account. Other aspects of my PhD research included causal inference with application to acoustic signal processing tasks and Bayesian methods for robot audition, where the latter was primarily tackled within the Two!Ears research consortium.